In the ever-evolving landscape of digital marketing, the role of Conversational AI in content recommendations has emerged as a game-changer. As businesses strive to create personalised and engaging experiences for their audience, harnessing the capabilities of Conversational AI has become imperative. This text delves deep into the nuances of Conversational AI, exploring its impact on conversion optimisation and content recommendations.
Understanding Conversational AI
Before we dive into its role in content recommendations, let’s establish a clear understanding of conversational AI. Conversational AI refers to the use of artificial intelligence in creating natural and dynamic interactions between humans and machines. Through technologies like chatbots and virtual assistants, businesses can now engage with their audience in real-time, providing instant solutions and recommendations.
The Psychological Aspect of Personalisation
Effective content recommendations rely heavily on the principle of personalisation, a fundamental concept grounded in the intricacies of consumer psychology. At its core, personalisation acknowledges and responds to the innate human desire for experiences that align seamlessly with individual preferences and needs. This crucial aspect of consumer engagement has been significantly enhanced with the integration of Conversational AI, pushing the boundaries of personalisation to new heights.
Conversational AI goes beyond the conventional understanding of user preferences by delving into the intricacies of user behaviour, preferences, and historical interactions. By harnessing advanced algorithms and machine learning, these systems meticulously analyse vast datasets to gain insights into each user’s unique digital footprint. This multifaceted understanding enables AI to discern patterns, anticipate preferences, and ultimately tailor content recommendations with an unparalleled level of precision.
The foundation of this process lies in the comprehensive examination of user behaviour. Conversational AI actively observes how users interact with content, identifying patterns in their choices, consumption habits, and engagement frequency. Through continuous learning and adaptation, the AI evolves its understanding, refining its ability to predict what resonates most with each individual.
Furthermore, the incorporation of user preferences adds another layer of complexity to the personalisation process. Conversational AI not only takes into account explicit preferences expressed by users but also employs sophisticated techniques to infer implicit preferences. This holistic approach ensures that content recommendations extend beyond surface-level interests, delving into nuanced aspects of user taste and preferences.
Historical context serves as a crucial dimension in this personalisation journey. By analysing a user’s past interactions, Conversational AI gains insights into their evolving preferences over time. This historical perspective allows the AI to offer recommendations that align not only with current interests but also with the user’s evolving tastes, creating a dynamic and adaptive content ecosystem.
The culmination of these personalised content recommendations is a user experience that transcends the transactional nature of content consumption. It fosters a profound sense of connection and understanding between the user and the platform, as the content suggested resonates authentically with the individual’s unique preferences. This connection, rooted in an AI-driven understanding of user intricacies, establishes a more meaningful and engaging relationship, driving long-term user loyalty and satisfaction.
Creating Captivating Leads Through Dynamic Conversations
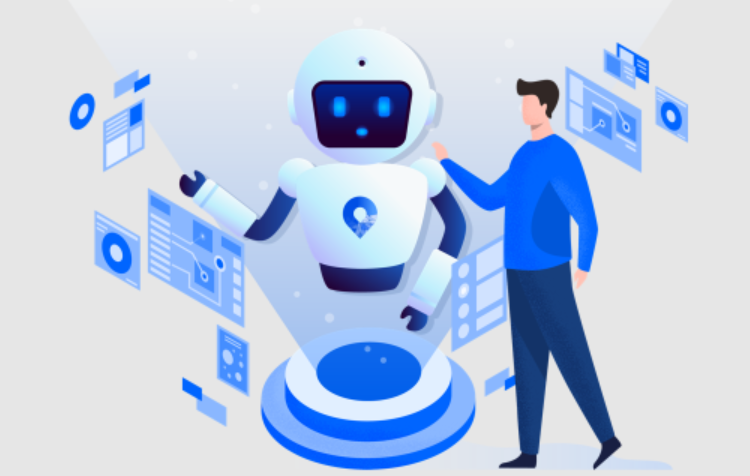
The journey of user engagement extends beyond the initial attraction generated by a compelling headline; the subsequent crucial element is the lead. In this intricate dance of captivating the audience, Conversational AI emerges as a transformative force, introducing a dynamic dimension to conversations that remarkably mirrors human interactions. Departing from static leads, businesses now have the ability to craft interactive and engaging dialogues that dynamically adapt based on user responses, fostering a level of interactivity that goes beyond traditional approaches.
Conversational AI, at its core, redefines the narrative by allowing businesses to create dialogues that evolve in real-time based on user input. This adaptability ensures that the user experience is not a one-way street but rather a responsive and personalised interaction that aligns seamlessly with the user’s preferences and needs. Unlike conventional static leads, which may become stale over time, the dynamic nature of Conversational AI keeps users actively engaged, contributing to a sustained level of interest and, consequently, an increased likelihood of conversion.
The interactivity facilitated by Conversational AI is a game-changer in the realm of user engagement. It transforms the user’s journey from a passive reading experience to an active and participatory dialogue. As users respond to prompts or queries, the AI dynamically adjusts the conversation flow, tailoring the content to match the user’s specific interests and intentions. This real-time adaptation not only captures and sustains user attention but also creates a sense of involvement and investment in the interaction.
The Art of Content Recommendations: Algorithms and Insights
Central to the transformative impact of conversational AI in shaping content recommendations is the intricate interplay of sophisticated algorithms. These algorithms, residing at the very core of AI-driven systems, transcend the confines of basic user data analysis. Instead, they operate on a multi-faceted approach, delving into nuanced factors such as user intent, context, and real-time behaviour to sculpt a personalised and dynamic content recommendation experience.
The essence of these algorithms lies in their ability to navigate the complexities of user interaction. Rather than relying solely on static user information, they actively consider the dynamic nature of user intent — deciphering not just what the user is interested in but also the underlying motivations and objectives guiding their content consumption journey. This deep understanding allows conversational AI to move beyond generic recommendations, providing a level of specificity that resonates with the user’s evolving interests and preferences.
Contextual awareness stands out as another pivotal dimension that these sophisticated algorithms masterfully navigate. Conversational AI goes beyond the surface-level understanding of user behaviour by taking into account the context in which interactions unfold. This contextual awareness ensures that content recommendations are not only relevant to the user’s general preferences but are also finely attuned to the specific circumstances or scenarios at play. The result is a content recommendation system that not only reflects the user’s preferences but also adapts seamlessly to the context in which they find themselves.
Real-time behaviour adds yet another layer of complexity to the algorithmic prowess of conversational AI. By continuously learning and adapting in real-time, these algorithms stay attuned to the user’s evolving patterns of interaction. This adaptability ensures that content recommendations remain aligned with the user’s journey, providing a dynamic and responsive experience. Whether the user’s interests shift, their preferences evolve, or their engagement patterns change, the conversational AI algorithms evolve in tandem, ensuring a consistently relevant and personalised content recommendation landscape.
The perpetual process of learning and adaptation defines the dynamic nature of conversational AI. Through constant refinement based on user interactions, these algorithms fine-tune their understanding, staying ahead of shifting user preferences. This evolutionary aspect ensures that content recommendations are not static but rather dynamic, mirroring the fluidity of the user’s journey and delivering suggestions that resonate authentically with their current interests and needs.
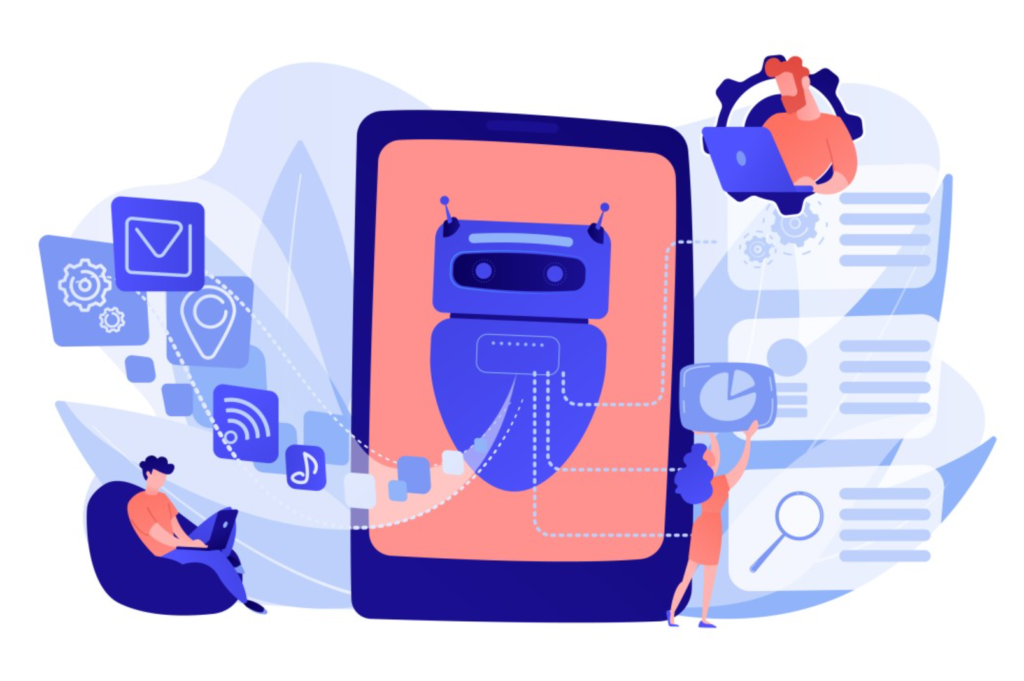
Persuasive Calls to Action: The Final Push
No conversion optimisation strategy is complete without a compelling call to action (CTA). Conversational AI can be leveraged to craft persuasive CTAs that feel natural and contextual. By understanding user preferences and behaviour, AI-powered CTAs can nudge users towards the desired action.
Challenges and Solutions
While the benefits of leveraging conversational AI in content recommendations are evident, businesses must also navigate a landscape riddled with challenges. Awareness and strategic planning are crucial as organisations seek to implement this transformative technology effectively.
One common hurdle in the integration of conversational AI revolves around user privacy concerns. As these systems actively collect and analyse user data to enhance personalisation, there is a growing need for businesses to address and mitigate potential privacy apprehensions. To overcome this challenge, transparency is key. Businesses should clearly communicate their data usage policies, providing users with comprehensive information about how their data will be utilised and assuring them of stringent security measures. Implementing robust encryption and anonymisation techniques can further bolster user trust, ensuring that the advantages of conversational AI are not compromised by privacy uncertainties.
Fine-tuning algorithms for accuracy poses another significant challenge. While conversational AI algorithms excel at learning and adapting, achieving optimal accuracy requires continuous refinement. The dynamic nature of user interactions demands ongoing adjustments to maintain relevance. To address this challenge, businesses should establish iterative testing and optimisation processes. Regularly evaluate the performance of algorithms, incorporating user feedback and behavioral data to identify areas for improvement. This iterative approach ensures that algorithms evolve in tandem with changing user preferences, enhancing the precision of content recommendations over time.
Interoperability and integration with existing systems represent additional hurdles. Businesses often grapple with seamlessly integrating conversational AI into their existing infrastructure, leading to compatibility issues and operational disruptions. To overcome this, a phased implementation strategy is advisable. Gradually introduce conversational AI features, allowing for thorough testing and adaptation. Collaborate with IT teams to ensure a smooth integration process, and choose platforms that offer robust APIs to facilitate compatibility with existing systems.
Language understanding and context awareness remain ongoing challenges in the field of conversational AI. While advancements have been made, achieving a truly natural and contextually rich conversation remains a complex task. Businesses can address this challenge by investing in ongoing research and development, staying abreast of the latest advancements in natural language processing and contextual understanding. Continuous training of conversational AI models with diverse datasets is essential to enhance language comprehension and context sensitivity.
Lastly, maintaining a balance between automation and human touch is a challenge often faced by businesses employing conversational AI. While automation streamlines processes, an excessive reliance on it may lead to a loss of the human touch. Striking the right balance involves designing conversational AI interfaces that seamlessly transition between automated responses and human intervention when necessary. Providing users with the option to escalate to human support ensures a personalised and empathetic experience when automated responses fall short.
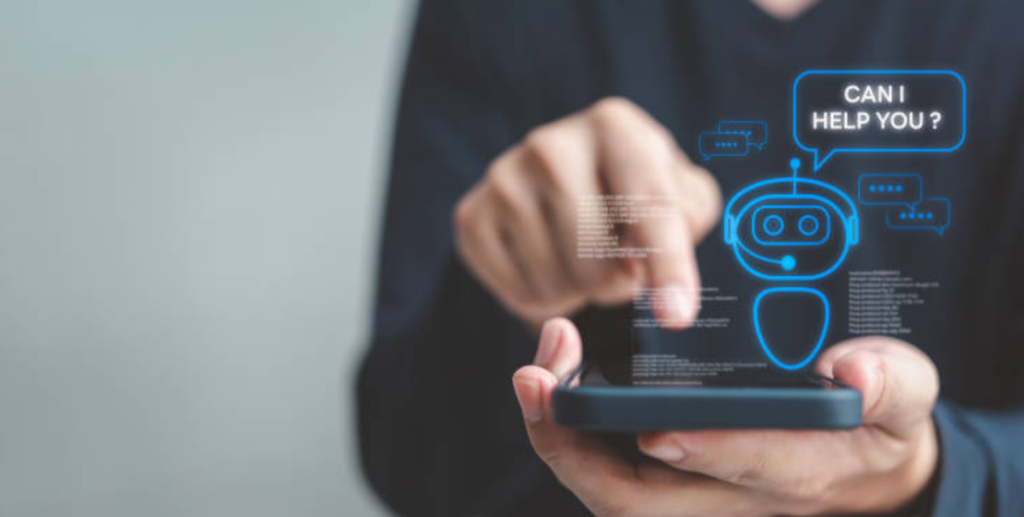
The Future of Conversational AI in Marketing
As technology continues its relentless march forward, the future of conversational AI in marketing presents a landscape filled with exciting possibilities and transformative trends. Staying attuned to these emerging developments is paramount for businesses seeking not only to keep pace with the evolving marketing ecosystem but to carve out a competitive edge in the digital realm.
One of the most prominent trends shaping the future of conversational AI in content recommendations is the relentless pursuit of enhanced personalisation. As algorithms become more sophisticated and capable of processing vast datasets, the focus shifts towards delivering hyper-personalised content experiences. Future conversational AI systems are poised to decipher even subtler nuances in user behaviour, preferences, and intent, offering recommendations that are not just relevant but deeply resonate with the unique tastes and motivations of each individual user. This heightened level of personalisation is expected to foster stronger connections between users and content, amplifying user satisfaction and loyalty.
Integration with emerging technologies is another frontier that holds great promise for conversational AI in content recommendations. As augmented reality (AR) and virtual reality (VR) technologies gain traction, businesses are exploring ways to seamlessly blend conversational AI with these immersive experiences. Imagine a scenario where users can engage in dynamic, AI-driven conversations while navigating augmented environments or receive personalised content suggestions within virtual spaces. This integration has the potential to elevate user engagement to new heights, creating immersive and memorable experiences that extend beyond the traditional boundaries of content consumption.
Voice-activated interfaces represent yet another frontier for conversational AI. As smart speakers, virtual assistants, and voice-activated devices become ubiquitous, the role of conversational AI in delivering content recommendations through voice commands is set to expand. Businesses can anticipate users interacting with content seamlessly using natural language, making content discovery more intuitive and accessible. This trend not only enhances user convenience but also opens up new avenues for brands to engage with their audience through voice-enabled interactions.
The integration of predictive analytics and machine learning algorithms is a trend that will further refine the predictive capabilities of conversational AI. By leveraging historical data, user behaviour patterns, and external factors, these advanced algorithms can anticipate user preferences and content needs more accurately. This predictive element ensures that content recommendations are not just reactive but proactive, staying one step ahead of user expectations and delivering suggestions that align seamlessly with their evolving interests.
Moreover, the evolution of multi-modal conversational AI is on the horizon. Future systems are likely to combine text-based interactions with visual and auditory components, enabling a more comprehensive and engaging conversational experience. This multi-modal approach opens up new avenues for creativity in content delivery, allowing businesses to leverage a diverse range of communication channels to connect with their audience in ways that are both innovative and immersive.